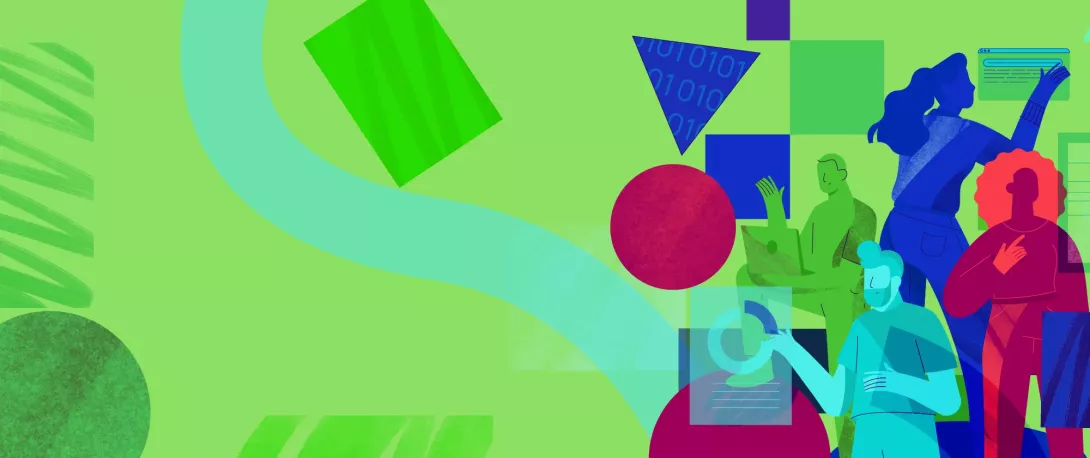
Much of the interest on AI and work concerns it possible effects on job losses – will jobs be replaced by AI or will they be transformed? While it is not possible to predict the future – particularly as the technology is still evolving – ILO researchers first developed a methodology in 2023, and later refined it in 2025, to estimate the potential effects of generative AI on existing occupations, and then in a second step, on employment.
The figure below shows the results of the analysis, which was based on a global assessment of the 436 detailed occupations that comprise the International Standard Classification of Occupations.
Select one of the occupations major groups to filter the occupations.
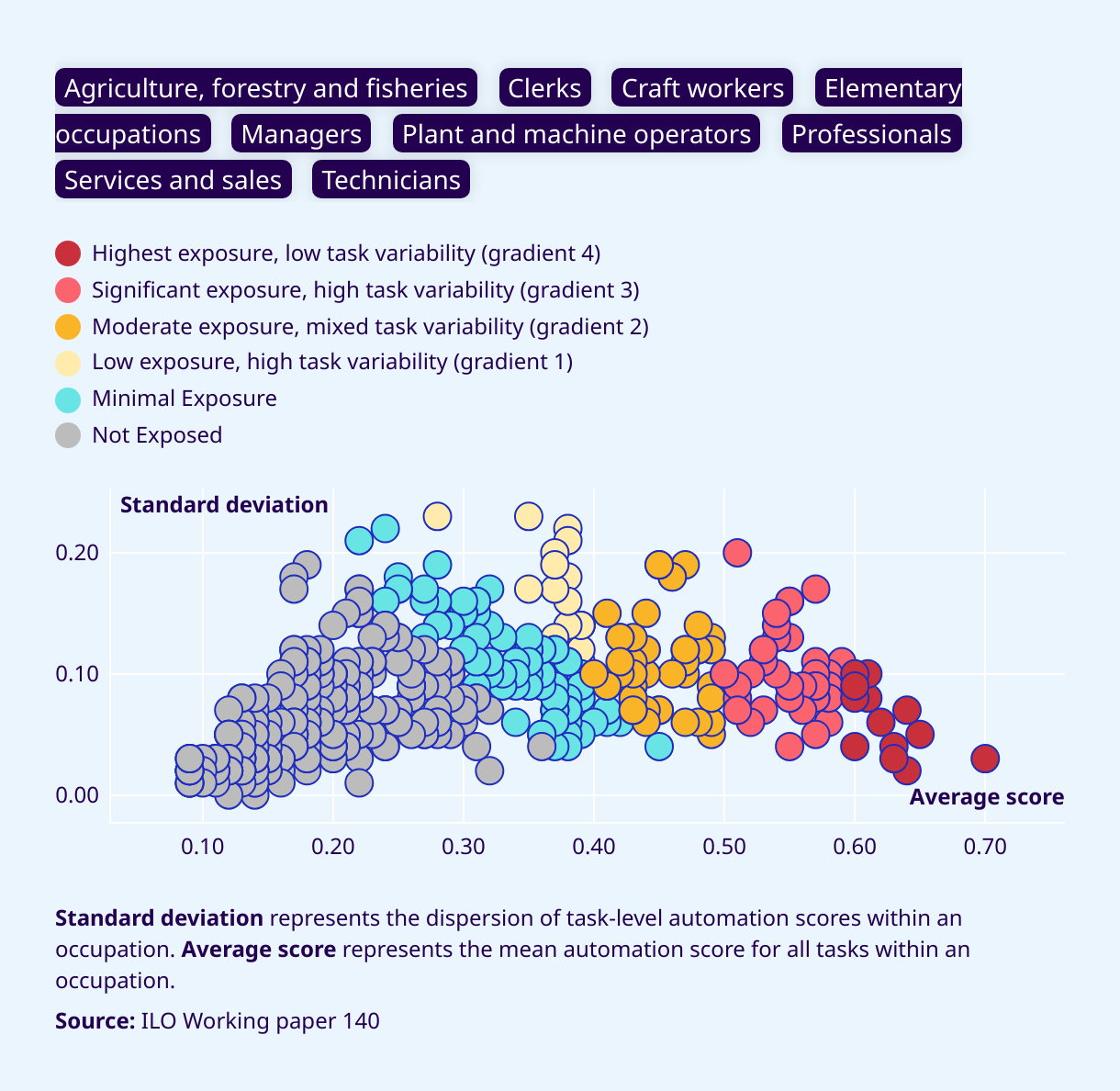
Each occupation is made up of tasks. Each of the tasks within an occupation received a 0-1 potential automation score, with 0 indicating it is not possible and 1 indicating it was entirely possible to perform the task with Generative AI.
The figure plots these occupations according to its average score (mean of all tasks within an occupation) and its standard deviation (the dispersion of task-level automation scores within an occupation).
The intersection of these scores was used to elaborate a framework for classification based on the following categories:
- Exposed: Gradient 4 (Highest exposure, low task variability): High and consistent GenAI exposure across tasks within the occupation. Most current tasks in these jobs have a high potential of automation, with little variability in task-level exposure.
- Exposed: Gradient 3 (Significant exposure, high task variability): Above-moderate occupational exposure: even though some tasks remain less exposed, the overall potential of automation of the current tasks with GenAI is growing in these occupations.
- Exposed: Gradient 2 (Moderate exposure, high task variability): Moderate occupational AI exposure, with high task-level variability. These occupations include a mix of some tasks that are exposed to GenAI and others not at risk, making the impact uneven.
- Exposed: Gradient 1 (Low exposure, high task variability): Low overall GenAI exposure at the occupational level, but high variability across tasks. Some tasks within these occupations have an elevated automation potential, even if the occupation as a whole remains strongly reliant on tasks that have a low potential of automation.
- Minimal Exposure (Low exposure, moderate task variability): Occupations with low GenAI exposure, where some tasks show moderate automation potential, but overall occupational exposure remains limited.
- Not Exposed: Occupations where most tasks remain relatively unaffected by GenAI, with low task variability and a stable low mean exposure score.
Overall, the findings indicate that few jobs consist of tasks that are fully automatable with current GenAI technology; nearly all occupations have some tasks that require human input. Gradients 1-3 comprise occupations where the automation of specific tasks is limited, and some degree of task variability exists.
What is the possible effect of generative AI on employment?
To assess how many existing jobs could be potentially affected, we apply these scores of occupational exposure to labour force survey data for more than 140 countries. This allows us to derive global and regional estimates as well as estimates among countries in different income brackets.
Percentage of jobs potentially affected by Generative AI, by income group
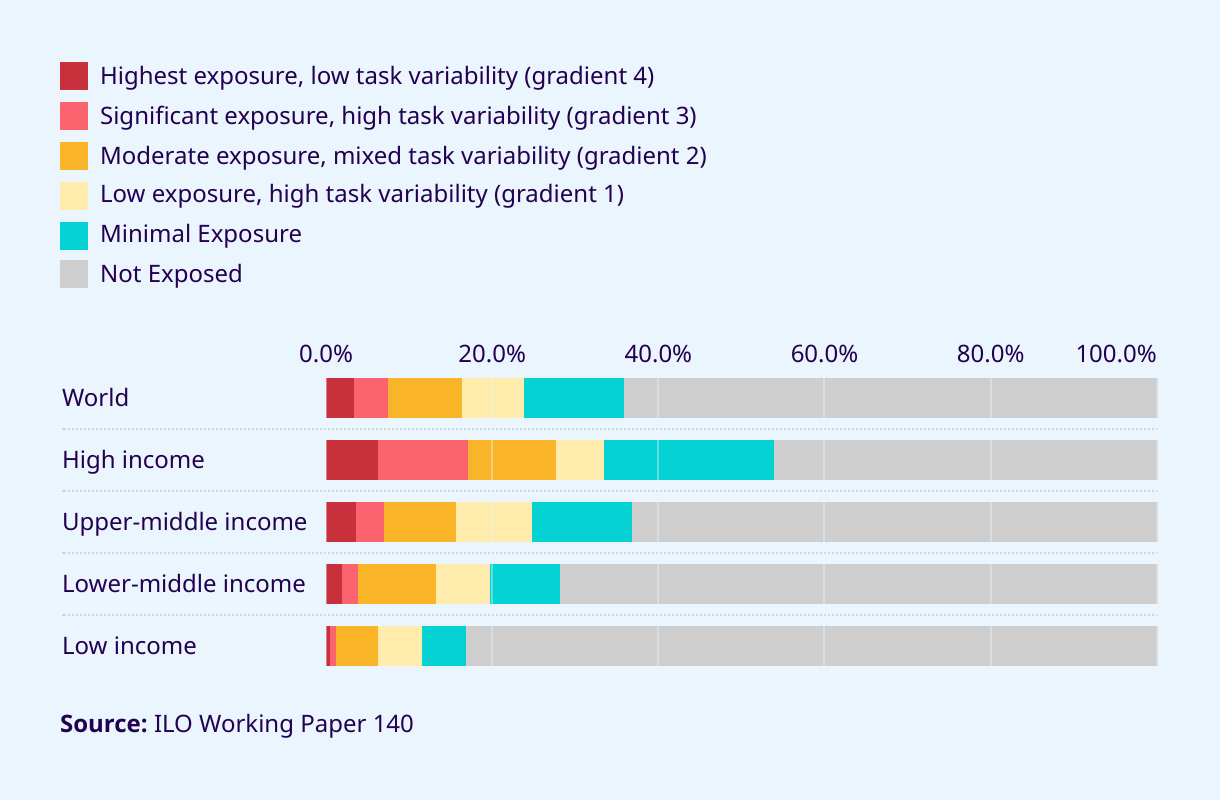
Percentage of jobs potentially affected by Generative AI, by region
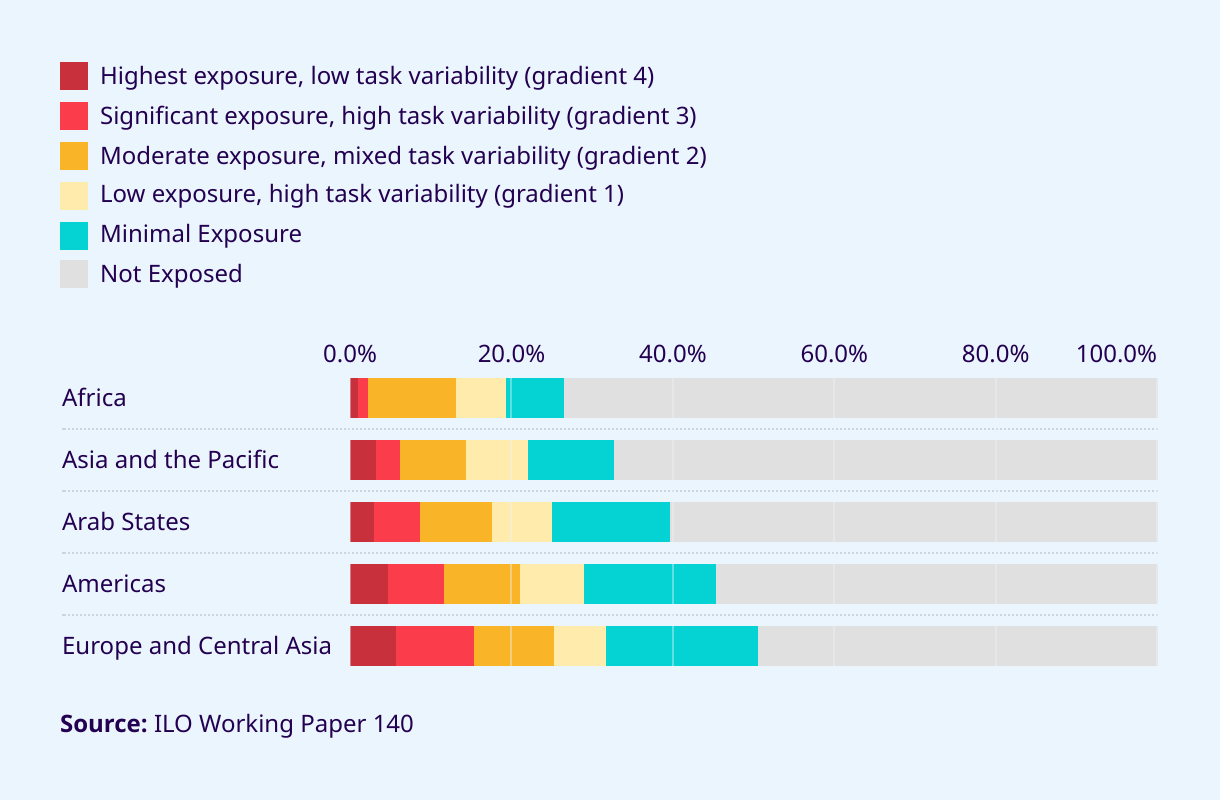
At the global level, about a quarter of all employment falls into one of the four exposure gradients, with significant differences between female and male employment, particularly in the top two exposure gradients. Female employment is also more concentrated in the top two exposure gradients, with 5.7% of female employment in gradient 3 and another 4.7% in gradient 4.
High-income countries have the highest share of employment in one of the four exposure gradients (34%). The total share of exposed employment declines significantly as income levels decrease, reaching just 11% in low-income countries.
These estimates reflect “potential exposure” to GenAI and not the actual impact. They represent the upper threshold of the percentage of employment that could be affected if GenAI technology were fully implemented. Infrastructure constraints (electricity, broadband), insufficient digital skills, the cost of technology, and inherent operational difficulties associated with the technology are just some of the barriers to full adoption.
As most occupations consist of tasks that require human input, transformation of jobs is the most likely impact of GenAI. Such a transformation is best managed through social dialogue.. Linking our refined index with national micro data enables precise projections of such changes, offering a foundation for social dialogue and targeted policy responses.